Navigating AI in Education with AI Literacy
DELTA Faculty Fellow and Associate Professor Paul Fyfe Shares His AI Perspective
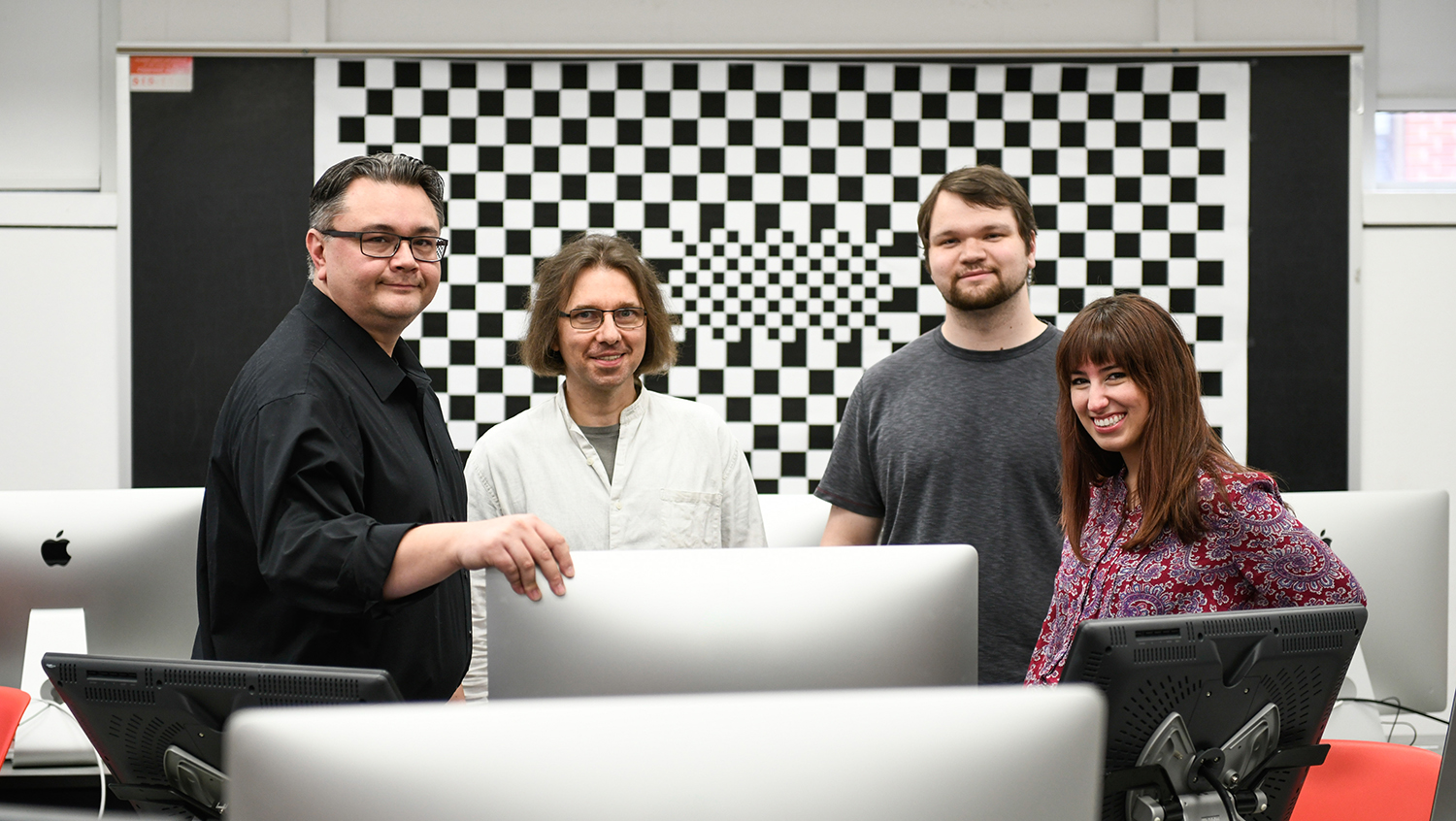
The rapid rise of generative AI has sparked intense debate in academia about its integration, limitations, ethics, and roles in education and industry. Its potential impacts have prompted scholars and institutions to emphasize the importance of developing comprehensive AI literacy.
Importantly, AI literacy is not just for students. In my own discipline of English, a taskforce on the topic recently advocated for “improving the AI literacy of all agents in the academic enterprise: students, faculty members, programs or departments, and institutions.” In other words, AI literacy is for all of us.
While we often associate “literacy” with a language or with reading and writing, the term has been adapted in response to major shifts in our technological landscape. For example, frameworks for information literacy emerged after widespread computerization in the 1970s and 1980s. When that landscape changed in the 1990s with personal computing and the World Wide Web, new forms of visual, multimodal, spatial and technological literacy (multiliteracies) were proposed. Then, in the 2010s, data literacy followed the advent of social media, smartphones and the surveillance economy they powered. Generative AI crashed into public consciousness in the 2020s and AI literacy wasn’t far behind.
All of these frameworks share two basic commitments. First, they combine applied skills with critical understanding. Their “literacy” is not simply how to use or navigate a given technology, but how to understand and help steer its broader social, economic, cultural and environmental consequences. Second, these literacies are all interdisciplinary. Like the technologies themselves, these new literacies cross traditional academic boundaries, demanding integrated knowledge and collaboration to address large-scale socio-technological change.
So, what comprises AI literacy? Various frameworks have been proposed for different educational levels. Those looking for a primer on K-12 might consider committee guidelines from the North Carolina Department of Public Instruction. In the context of higher education, I would recommend the “adjustable interdisciplinary socio-technical curriculum” by Sri Yash Tadimalla and Mary Lou Maher at UNC Charlotte. Their proposal usefully lays out key knowledge outcomes and suggests how it might be adapted given any institution’s curricular constraints. Their “four pillars of AI Literacy” offers a general need-to-know for everyone — including students, administrators, staff and instructors — are as follows:
1. Understanding the scope and technical dimensions of AI.
In other words, how it’s made, by whom, for what purposes and with what material impacts. AI covers a huge range of technologies and methods, but the recent surge of public interest and private investment has mostly focused on language models including GPTs. The scale and complexity of these models may seem intimidating, but — take it from an English professor — their basic operations are still understandable. (For starters, try this illustrated piece from the Financial Times.)
2. Learning how to interact with Gen-AI in an informed and responsible way.
This includes understanding what it can and cannot do, its strengths and weaknesses, etc. The phrase “artificial intelligence” seems to claim superhuman capacities that work independently of our input — and may even surpass us. Yet this is more a legacy of science fiction than a reality of predictive machine learning. The limitations of generative AI are increasingly well documented, including false information, monocultural thinking, cultural biases, its effects on critical thinking, etc. More research is also emerging about its capacities for creative assistance and support in certain contexts.
3. Critically reviewing the issues of ethical and socially responsible AI in learning/work environments.
As scholars like Timnit Gebru have pointed out, big tech is the only major industry that does not have to prove the safety of its products before release. As a result, the deployment of AI has far outpaced regulatory frameworks or normative standards for its use. This includes unresolved questions about the deskilling of labor in various industries, escalating energy demands of data centers, disinformation, the risks of agent-based AI and changing social dynamics in AI-powered communication and education.
4. Social and future implications of AI.
The discourse around AI tends to emphasize its inevitability, as if we have no choice in the matter. However, we can and should envision the futures we want — or want to avoid. Speculative thinking about those futures, and how we can design and shape them, is an essential part of how we understand and interact with AI now.
Determining how to effectively incorporate AI literacies poses a significant challenge, particularly regarding its placement within educational curricula and the strategies needed to engage administrators, technologists, staff, and instructors who will influence or restrict the implementation of AI.
From my own perspective, NC State can become a leader in AI not by embracing the fanciest technologies, but by leveraging its human expertise and interdisciplinary strengths. This encompasses various initiatives that are already in progress, such as those at DELTA, the Data Science and AI Academy, the Friday Institute and the newly established interdisciplinary Center for AI in Society and Ethics (CASE), which has been supported by an NEH Humanities Research Centers grant on Artificial Intelligence. The future of artificial intelligence relies on people and should be built upon strong AI literacy throughout our university.
Interested in sharing your knowledge and learning from other instructors? Apply for a Faculty Fellows Grant during the next application cycle!